How Americans View the Economy’s Progress (or Lack Thereof)
September 17, 2024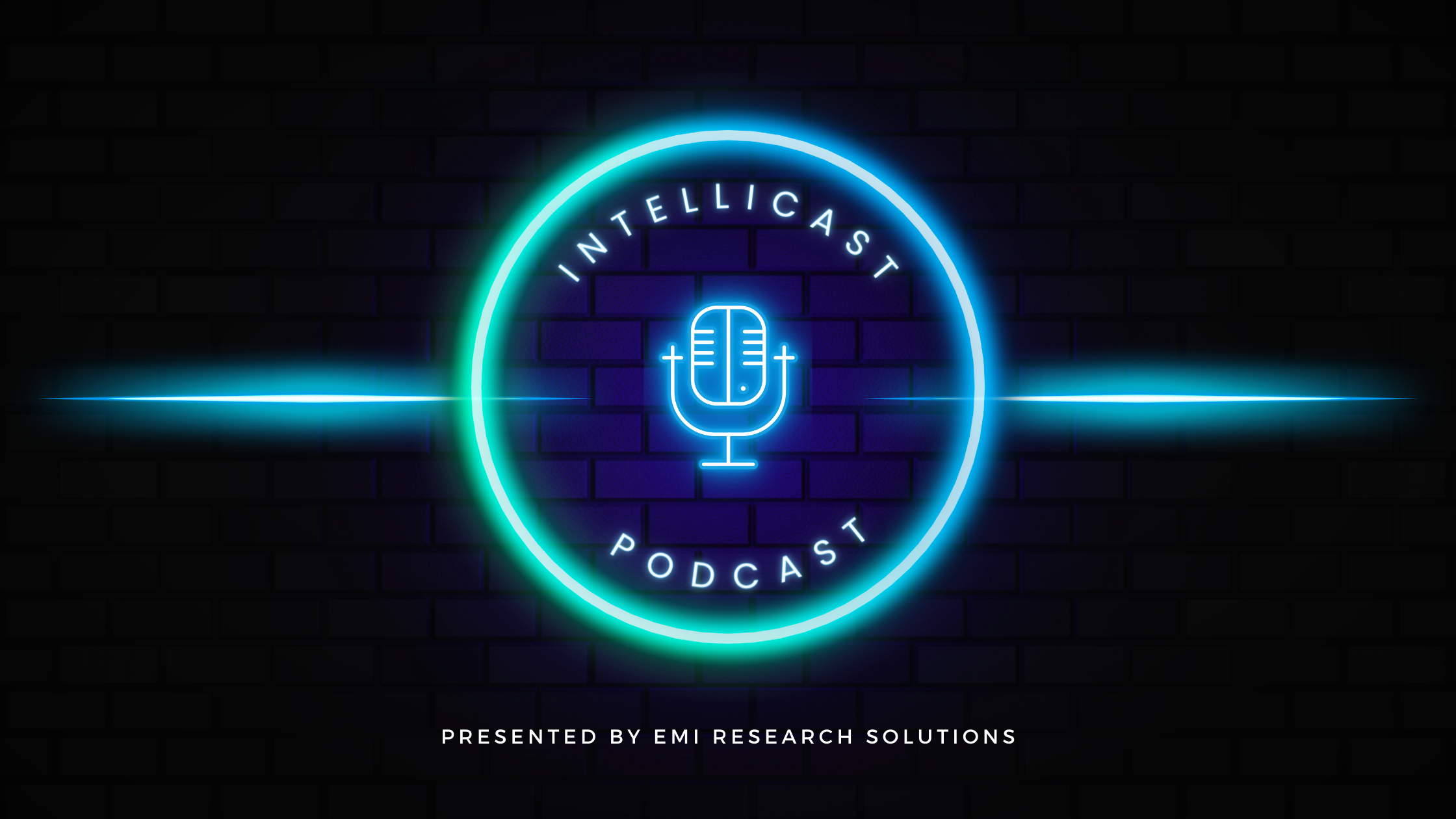
New AI Tools Everywhere
October 8, 2024Since the advent of large language models and generative AI, synthetic data and synthetic personas have been a hot topic in the market research industry. New companies have been popping up like weeds in a garden, offering better data than panel data based on synthetic profiles.
The thing is— synthetic personas have much more in common with online sample panels than you may think.
1. All Are Different
Stop me if you have heard this one – online panels are all different from one another. This is because of various factors, from recruiting methods, incentive structure, invite methodology, internal management, and more – all lead to panels (and their respondents) having different attitudes and behaviors.
Guess what? Synthetic personas are all different from one another. I don’t just mean that the Gen Z Jenna persona differs from the Boomer Brad persona. I mean that if two different companies have a Gen Z persona, they are different. There are three big reasons for the differences.
First, synthetic personas are built using different AI models or engines. Some companies utilize public LLMs like ChatGPT or Claude, while others use privately built LLMs. There are also the types of LLMs that are used as engines for their synthetic profiles. Some use censored models and others use uncensored models. Think about it this way: Have you ever gone to ChatGPT and asked it a question, then gone to another generative AI like Claude and asked the same question? Did you get the same answer? I bet you didn’t. These models are not all programmed the same, so you get different answers.
The second factor leading to synthetic personas being different is the data used to train them. Some personas are built using public data sources, others using proprietary data sources, each leading to differences in results. You could use two similar data sets to create two personas using the same AI model as the engine and then ask each the exact same question. You would end up getting different answers.
Finally, the third factor leading to synthetic personas being different is your prompts. You can build personas using the same AI engine and data set but get different answers depending on the prompts you use and how you ask the questions.
When you combine these three factors, synthetic personas are just as unique as panels.
2. They Change Over Time
Another major way that online panels and synthetic personas are similar is that they change over time. With panels, the attitudes and behaviors of panelists change over time. This can be due to changes in how the panel is managed, panelist turnover, new recruiting sources, merger or acquisition of the panel, or other factors. With synthetic personas, the AI models they are built from are constantly changing. You can barely go for a week without a news story about how the latest model of ChatGPT, Claude, Gemini, etc., has been released. That is because generative AI is designed to evolve over time. Unless you prompt it to remember its previous responses, it will generate new insights and answer with each new prompt.
Here is another way to think about it. You could have built a custom GPT using a data set and asked it a series of questions to get data. Then you can come back a couple of months later, use the same custom GPT, and ask the same questions, and you will get a different answer. That is because the AI model has been updated, and now it may be processing the information differently or considering the question differently than it did previously. This would be the same if you asked it the same question 10 times in a row as well.
As the use of synthetic personas starts to become a more significant part of the market research toolkit, it’s essential to recognize their evolving nature. Like online sample panels, they offer distinct insights and require careful handling. The key takeaway? Both synthetic personas and online panels have unique strengths, but neither should be viewed as static or one-size-fits-all. Embracing their complexity can lead to smarter, more informed decisions.