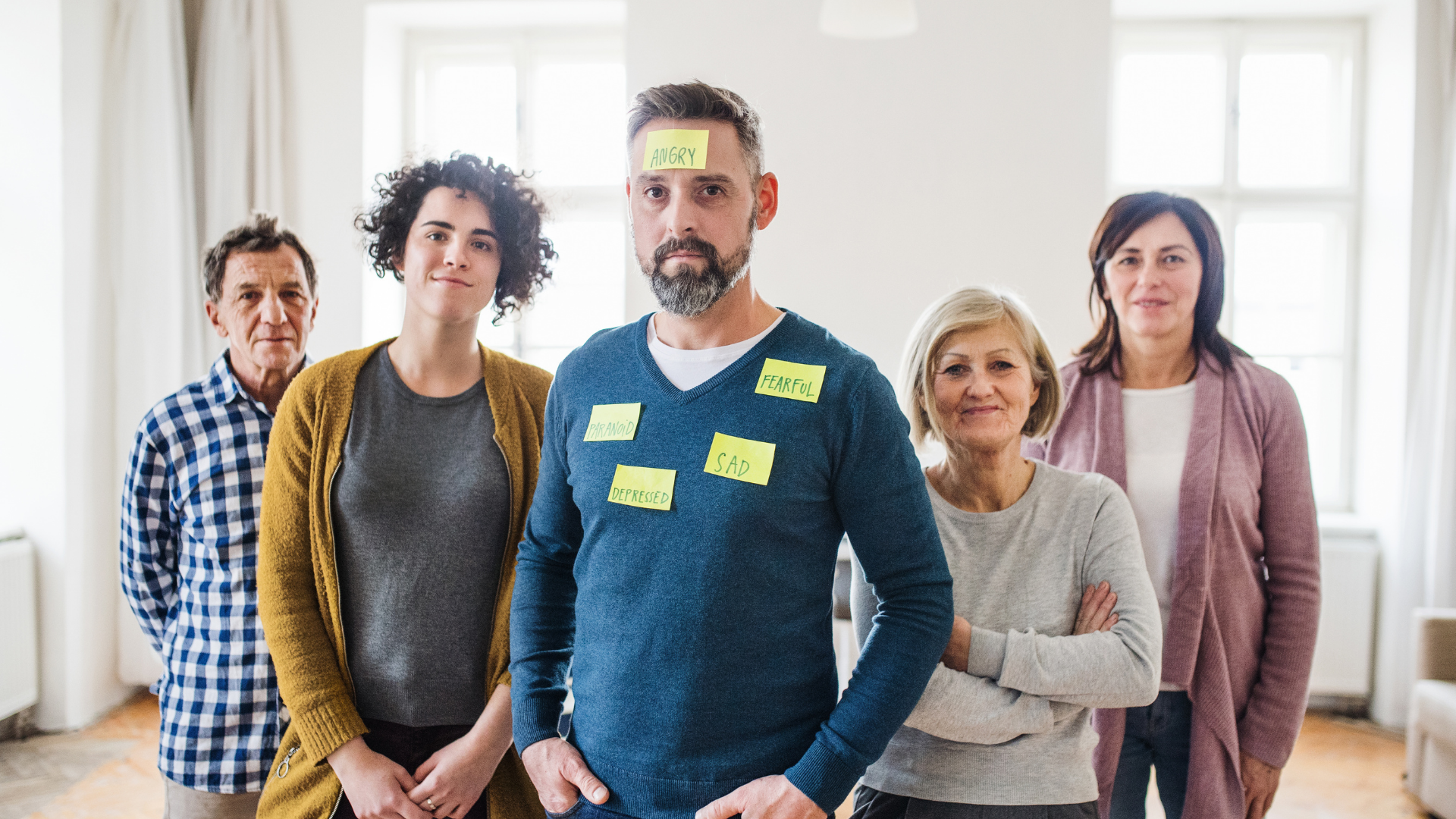
The Effects of Emotion on Brand Ratings and Awareness
June 28, 2021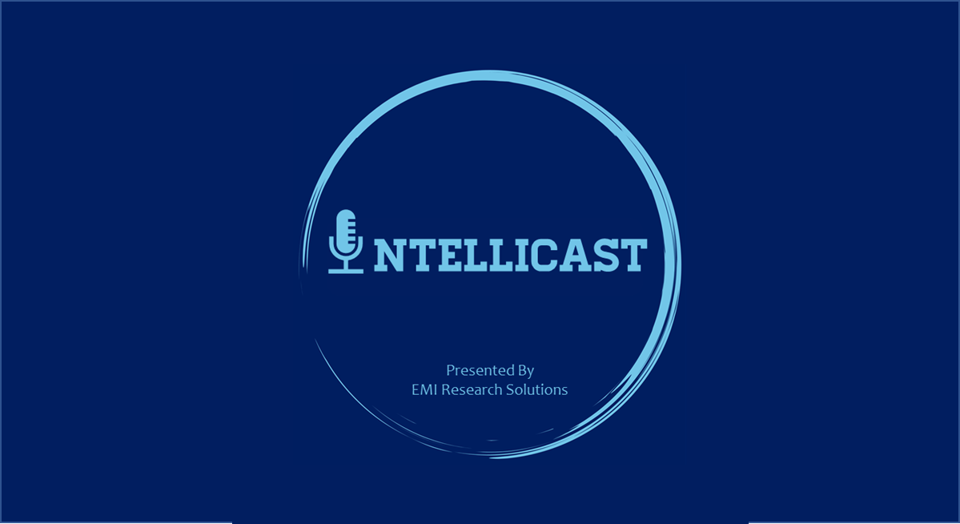
Intellicast S4E22 – Combining First and Third-Party Data and MRX News
July 1, 2021The number one question we must ask ourselves when planning a survey is, who am I targeting? Respondents must meet the criteria you’re looking for in order to give you the data you need. We accomplish this by adding screener questions to your survey. The screener is the part of the questionnaire where you identify your desired target audience. Just as survey design is crucial to data quality, so are screeners.
In this blog, we take a look at 5 best practices for using screener questions in your study.
1. Make Sure Your Survey Isn’t Leading
One of the first things you want to check for when creating a great screener is ensuring that the introduction of your survey is not leading, meaning it does not persuade the respondents to answer the questions a certain way by giving clues to the target audience you’re trying to reach.
Along the same lines, you don’t want any questions within your survey to be leading either. Having leading questions can cause many problems for your data quality. It can make it easier for fraudsters to be admitted into your survey, providing you with poor data. Even if the admitted respondents are not fraudsters, they may simply not be a member of your target audience, meaning they also provide poor data.
2. Avoid Certain Types of Questions
The first type of question you want to avoid is Yes/No questions. Yes/No screener questions tend to have a higher level of suggestibility, making them more susceptible to allowing fraudsters to enter your survey. Since there’s a 50/50 chance of getting them right, they’re easier to get past. Try sticking to multiple choice or other multiple answer options in your screener to make it more difficult for people outside of your target audience to get into the core part of your study.
The second type of question you want to avoid is double-barreled questions. These are questions that ask two things in a single question and can lead to inaccurate data because they don’t make it clear what is being asked of the respondents. Don’t be afraid to turn double-barreled questions into two questions.
3. Imagine There is No Targeting
When building a screener, it can be helpful to act as though there is no targeting built into the sample who receives your study. This will ensure that you draft your screener thorough enough that no fraudsters can enter your survey.
4. Ensure There is an Answer Option for Everyone
We’ve already discussed the necessity of using multiple choice questions in your screener. Within those questions, you want to ensure that you have an answer option for everyone, even if that answer is “Other” or “None of the Above.” Providing all respondents with options will help you weed out people outside of your target audience and avoid making your questions leading.
5. Watch Your Question Order
When you are planning your screening questions, the order they are presented to respondents can be a key difference between quality data and useless data. You don’t want to have responses to early questions affecting responses to later questions.
For example, many studies have quotas built into their sample plans to ensure they meet certain criteria. These criteria may be based on gender, region, age, and other demographics. These termination points are built in to remove people who do not fit the target audience. You want to make sure that the necessary termination points are at the beginning of your screener.
This generally revolves around respondent experience and ensuring that your surveys are a reasonable length. Having a short screener means that respondents who get disqualified will be less frustrated, and those that do qualify will not be fatigued before they enter the survey.
Screening questions are a critical factor in data quality and should not be overlooked throughout the survey development process. If you need help with your screening questions, reach out to us here. For more tips on Data Quality, check out the previous installments of our Data Quality 101 series:
Data Quality 101: De-Duplication